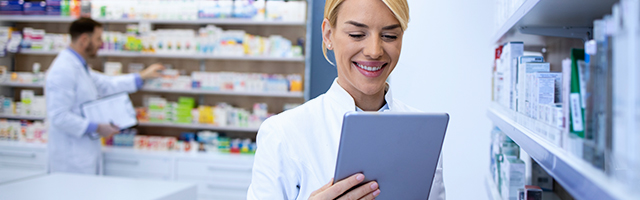
Conversational Analytics in Embedded Analytics: Benefits, Use Cases, Considerations
Software providers are under increasing pressure to increase customer engagement and enhance the value of their applications to meet the evolving needs of their customers. One of the most effective ways to achieve this is by integrating advanced analytics capabilities, such as conversational analytics, directly into their applications. This powerful tool allows end-users—your customers—to gain […]
Software providers are under increasing pressure to increase customer engagement and enhance the value of their applications to meet the evolving needs of their customers. One of the most effective ways to achieve this is by integrating advanced analytics capabilities, such as conversational analytics, directly into their applications. This powerful tool allows end-users—your customers—to gain actionable insights from their interactions, improving their decision-making and overall experience with your product.
Conversational analytics is not just another feature; it’s a transformative capability that can significantly elevate the user experience within your software. By embedding conversational analytics into your applications, you empower your customers to leverage natural language processing (NLP) and machine learning to gain deeper insights from their data. This article explores the benefits, use cases, and critical considerations for ISVs (Independent Software Providers) looking to integrate conversational analytics into their applications.
What is Conversational Analytics?
Conversational analytics refers to using Natural Language Processing (NLP) to ask questions about your data and receive answers and insights. For ISVs, this means enabling your application’s users to interact with their data through natural language, allowing them to distill meaningful insights without needing to be data experts.
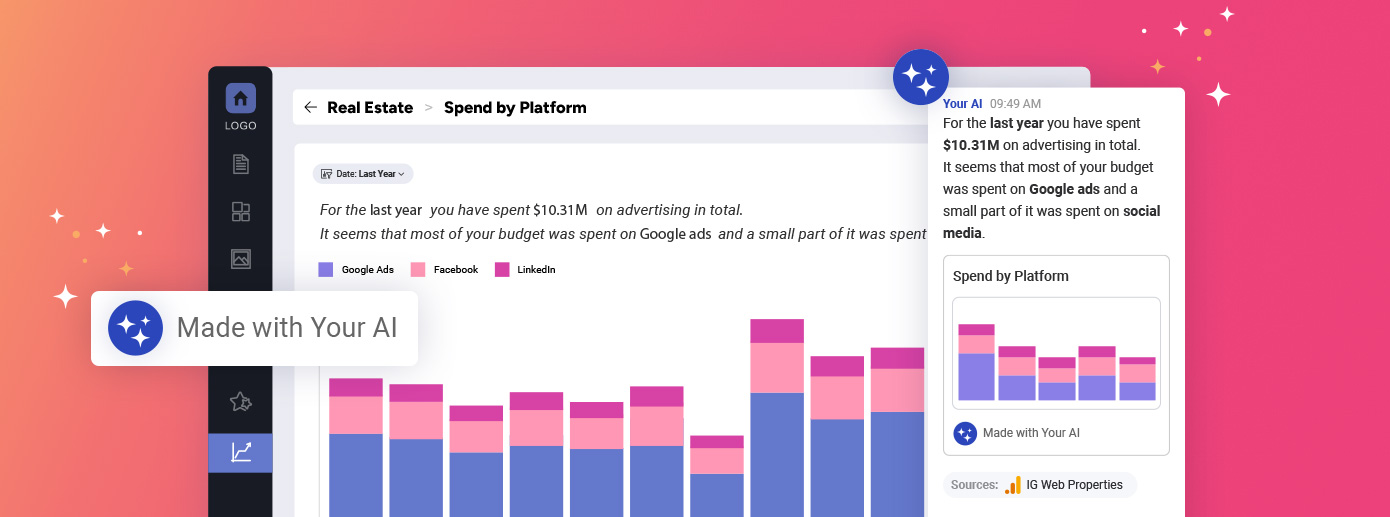
How Conversational Analytics Works
For software providers, embedding conversational analytics into their applications involves integrating Natural Language Processing (NLP) and machine learning algorithms directly into their platform. The system captures and processes these interactions in real-time, identifying trends, sentiments, and intents. The key advantage here is that the insights are seamlessly integrated into the user experience, providing immediate, actionable feedback without requiring users to leave the application or possess advanced data skills.
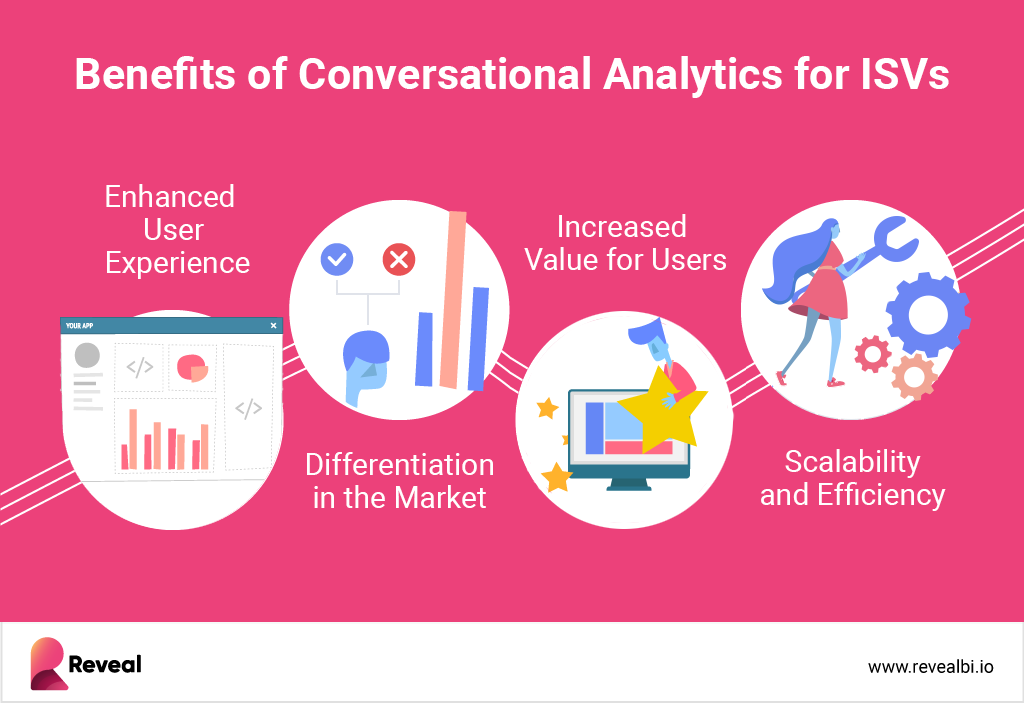
Benefits of Conversational Analytics for ISVs
By embedding conversational analytics into your applications, software vendors can unlock significant advantages that not only enhance the user experience but also differentiate your software in a competitive market.
Enhanced User Experience: By integrating conversational analytics into your application, you provide your customers with tools to better understand and engage with their data. This leads to a more personalized and satisfying user experience, which can increase customer loyalty and reduce churn.
Differentiation in the Market: Offering advanced analytics capabilities like conversational analytics can set your application apart from competitors. This differentiation can be a key selling point, attracting more users to your platform.
Increased Value for Users: The insights derived from conversational analytics can help your customers make more informed decisions, increasing the overall value they derive from your software. This, in turn, can lead to higher satisfaction and retention rates.
Scalability and Efficiency: Automating the analysis of user interactions not only provides immediate value to your customers but also helps your application scale more efficiently as the volume of interactions grows.
Conversational Analytics Use Cases in Embedded Analytics for ISVs
Embedding conversational analytics into your applications allows customers to extract valuable insights directly within their workflows. Here are some specific examples:
ERP Applications: In an Enterprise Resource Planning (ERP) system, users can leverage conversational analytics to ask questions like, “What are the current inventory levels for our top-selling products?” or “Which suppliers have the fastest delivery times over the last six months?” The system can instantly analyze data across various modules—such as inventory, procurement, and sales—providing users with real-time insights that help them optimize operations and make data-driven decisions.
Healthcare Applications: For healthcare providers using electronic health record (EHR) systems, conversational analytics can enable practitioners to query patient data in natural language. For instance, a doctor might ask, “What were the blood pressure trends for this patient over the past year?” or “Which patients are due for their annual check-up this month?” The system processes these queries and delivers actionable insights, helping healthcare professionals improve patient care and streamline administrative tasks.
Financial Management Tools: In a financial application, end-users could ask questions such as, “What was the monthly revenue growth last quarter?” or “Which expenses have increased the most year-over-year?” Conversational analytics can quickly parse through financial data, generating reports or visualizations that provide clear answers, enabling users to manage their finances more effectively.
Customer Relationship Management (CRM) Systems: Sales teams using CRM software could ask, “Which leads are most likely to close this week?” or “What is the average response time to customer inquiries?” The embedded analytics can sift through communications and sales data to identify patterns, helping sales representatives focus on the most promising opportunities and improve customer satisfaction.
Considerations for ISVs Implementing Conversational Analytics
When integrating conversational analytics into your applications, there are several key factors to consider ensuring a successful implementation:
Data Privacy and Security: As you enable your customers to collect and analyze conversational data, it’s crucial to ensure that this data is handled securely and in compliance with regulations such as GDPR.
Integration with Existing Systems: Ensure that conversational analytics integrates seamlessly with your application’s existing analytics and CRM systems, providing a cohesive experience for your users.
Scalability: As your user base grows, so too will the volume of conversational data. Choose a solution that can scale with your application without compromising performance.
User Training and Adoption: Consider how you will educate your customers on using conversational analytics effectively. Providing training resources can help ensure they maximize the value of this feature.
Conclusion
For ISVs, embedding conversational analytics into your applications is a strategic move that can significantly enhance the value provided to your customers. By enabling users to gain actionable insights from their interactions, you not only improve their experience with your product but also differentiate your software in a competitive market. As you consider integrating conversational analytics, keep in mind the benefits, use cases, and key considerations to ensure a successful implementation that meets the needs of your users.