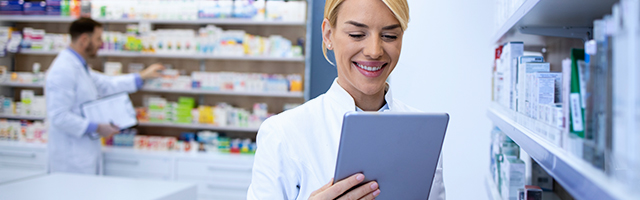
Embedded Analytics
As businesses continue to make analytics and BI their top investment priority, choosing the right embedded analytics vendor to partner with is paramount. But what exactly does embedded analytics do? What are its benefits and key elements? How to choose the best solution for your business needs? All of these are questions that we know a lot of you still ask yourself. Here, we’ve gathered everything that you need to know about embedded analytics.
Table of contents:
What Is Embedded Analytics?
Embedded analytics software delivers real-time reporting, interactive data visualization, and/or advanced analytics, including machine learning, directly into an enterprise business application. The data is managed by an analytics platform, and the visualizations and reports are placed directly within the application user interface (UI) to improve the context and usability of the data for business users.
What Is Embedded Analytics Used For?
Embedded analytics tools can be used in many different industries, allowing businesses to collect and analyze data for various purposes. The main usage of embedded analytics is to provide data and up-to-date business insights in the simplest way possible so that any user or application can use and act on them.
When you integrate analytics into your business applications, you can achieve many benefits, such as:
- View, edit, and create sales, KPI dashboards, and more directly from your app
- Provide dashboards in context with other features in your application
- Connect your different data sources for easy access
- Turn on and off features for your end users or customers
- Total control over the security and look and feel of the data in your app
- Customize actions based on your customers’ interaction with dashboards and visualizations
Organizations are taking advantage of these benefits by integrating embedded analytics into their web pages, business applications, commercial software products, and external portals
Additional Information:
The Difference between Embedded Analytics and Traditional BI
Benefits of Embedded Analytics